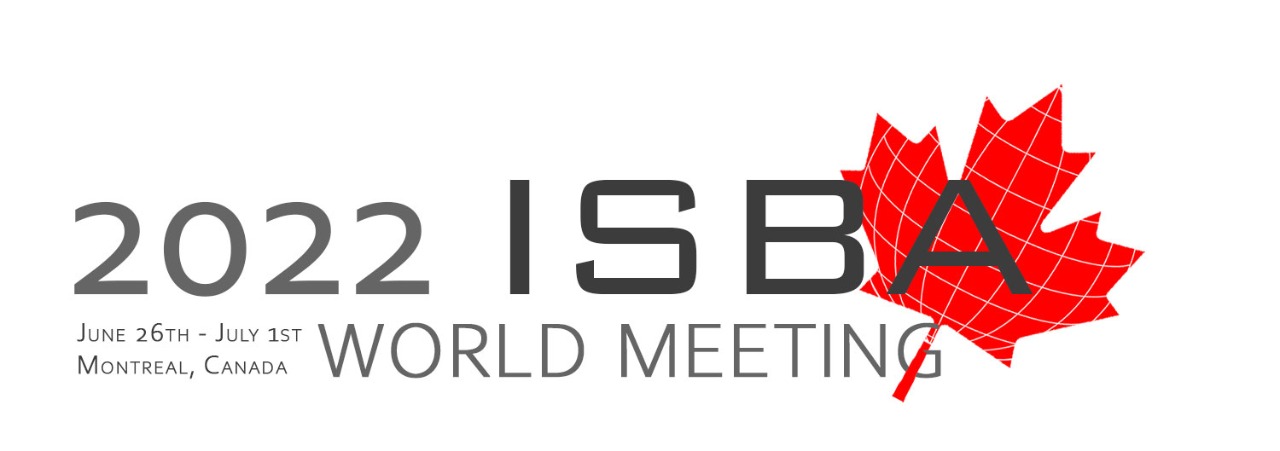
News
-
The presentations from ISBA are available here.
Click the following link to view all of the available presentations: ISBA Presentations
-
The Bruno de Finetti lecture is available to watch.
Click the following link to watch the Bruno de Finetti lecture: Bruno de Finetti Lecture
-
Conference photos are now available to view.
Click the following link to see photos from the conference: ISBA 2022 Montreal Photos
-
Guidance around COVID-19 and information on COVID-19 tests for attendees.
Some updated COVID-19 guidance from ISBA: COVID-19 Guidance
-
Create your conference agenda using Whova.
You can now access the ISBA 2022 program and plan your personal schedule using the Whova web portal or by downloading the Whova app.
-
The conference program is now available on Whova.
You can access the conference program here: Conference Program
-
The conference will take place fully on-site.
We are excited to welcome everyone back for a fully on-site conference. There will be no virtual option for this conference.
-
The impact of COVID-19 on the conference.
The conference will adhere to the follow the COVID-19 guidelines that are being enforced in Montreal, Canada. Some of the current guidances can be found here:
About The Event
In June 2022 Montreal will be the center of Bayesian thinking in the world as we celebrate the 30-year anniversary of ISBA. The purpose of the meeting is to bring together the diverse international community of investigators in statistics who develop and use Bayesian methods to share recent findings and to present new and challenging problems. Montreal is a city where the world comes to meet and share ideas, a city that attracts talent from around the world and is known for the warm welcome it extends to visitors.
Where
Hotel Bonaventure, Montreal, Canada
When
Sunday to Friday
June 26th - July 1st
Committees
The organizing and scientific committee members
- Radu V. Craiu (Univeristy of Toronto)
- Rob Deardon (Univeristy of Calgary)
- Aurélie Labbe (HEC Montréal)
- Geneviève Lefebvre (UQAM)
- Alexandra M. Schmidt (chair, McGill University)
- James V. Zidek (University of British Columbia)
Organizing Committee
- Lamiae Azizi (University of Sydney)
- Tamara Broderick (Massachusetts Institute of Technology)
- Ismael Castillo (Sorbonne Université)
- Arnaud Doucet (Oxford University)
- Ed George (The Wharton School of the University of Pennsylvania)
- Amy Herring (Duke University)
- Matthias Katzfuß (Texas A&M University)
- Yongdai Kim (Seoul National University)
- Thanasis Kottas (University of California, Santa Cruz)
- Fan Li (Duke University)
- Ramses Mena (National Autonomous University of Mexico)
- Omiros Papaspiliopoulos (Bocconi University)
- Matteo Ruggiero (University of Torino/Collegio Carlo Alberto)
- Alexandra M. Schmidt (McGill University)
- Mark Steel (University of Warwick)
- Botond Szabo (chair, Bocconi University)
Scientific Committee
Plenary Speakers
The list of speakers
Foundational Lectures
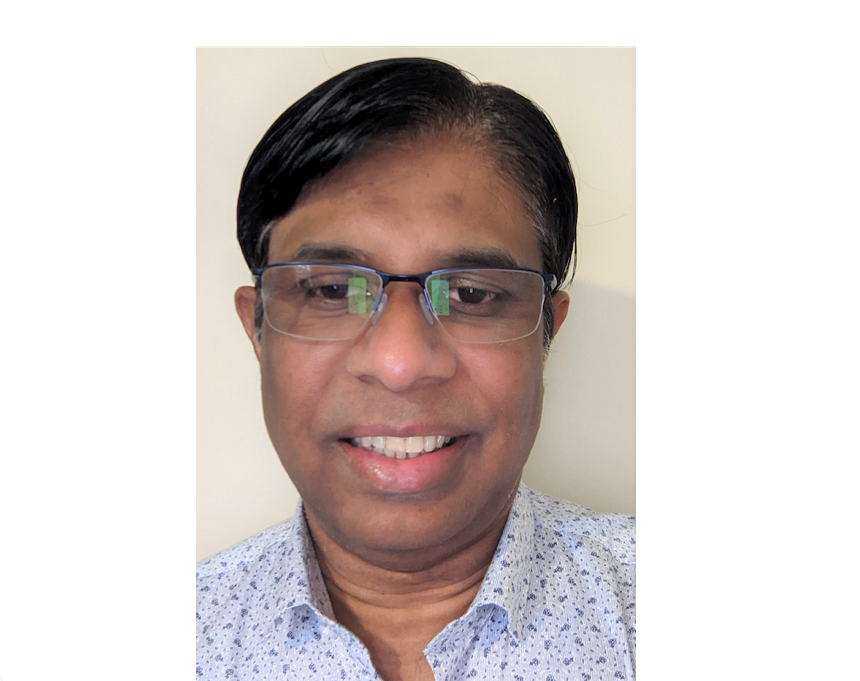
Subhashis Ghosal
North Carolina State University
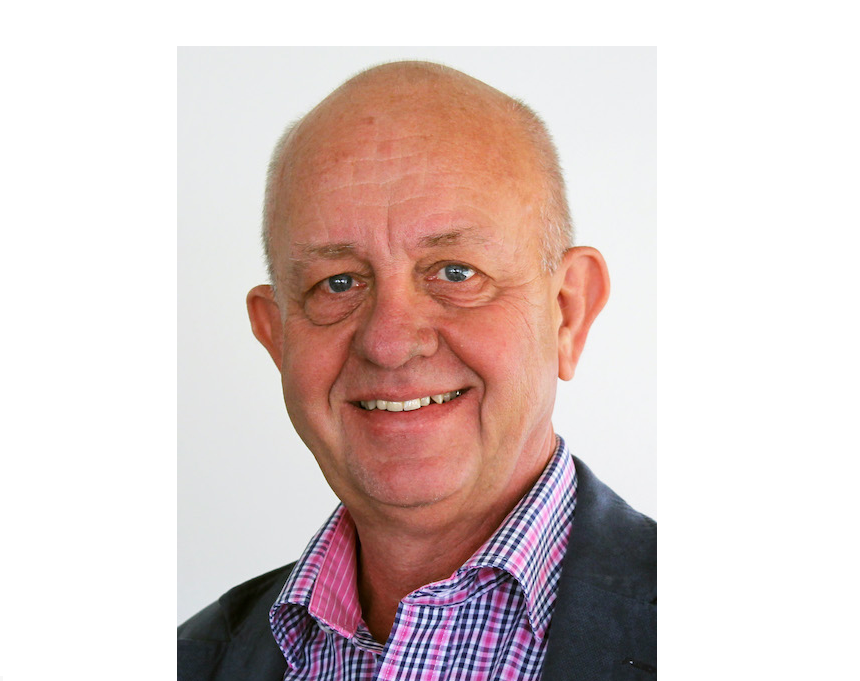
Steffen Lauritzen
Univeristy of Copenhagen
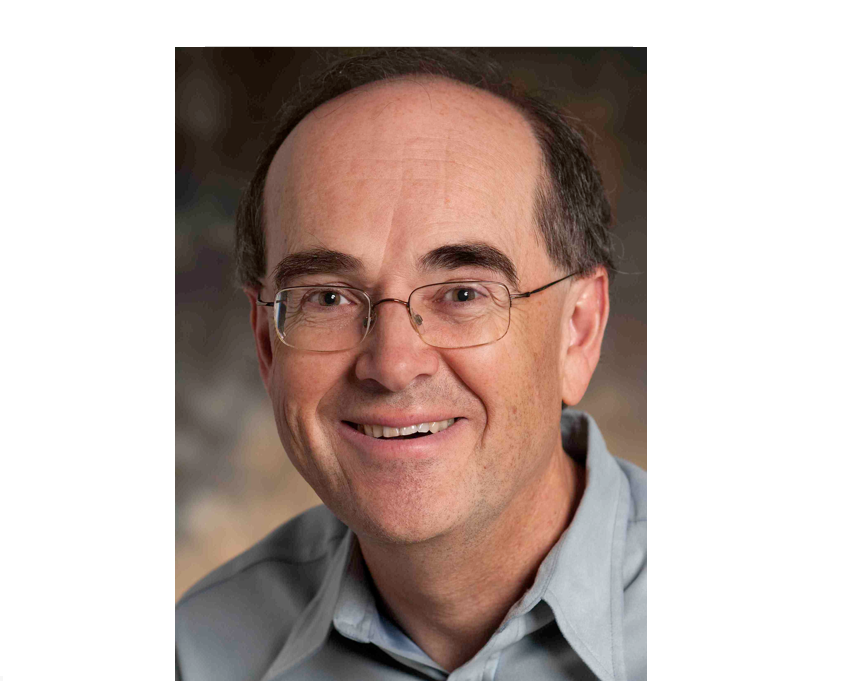
Adrian Raftery
University of Washington
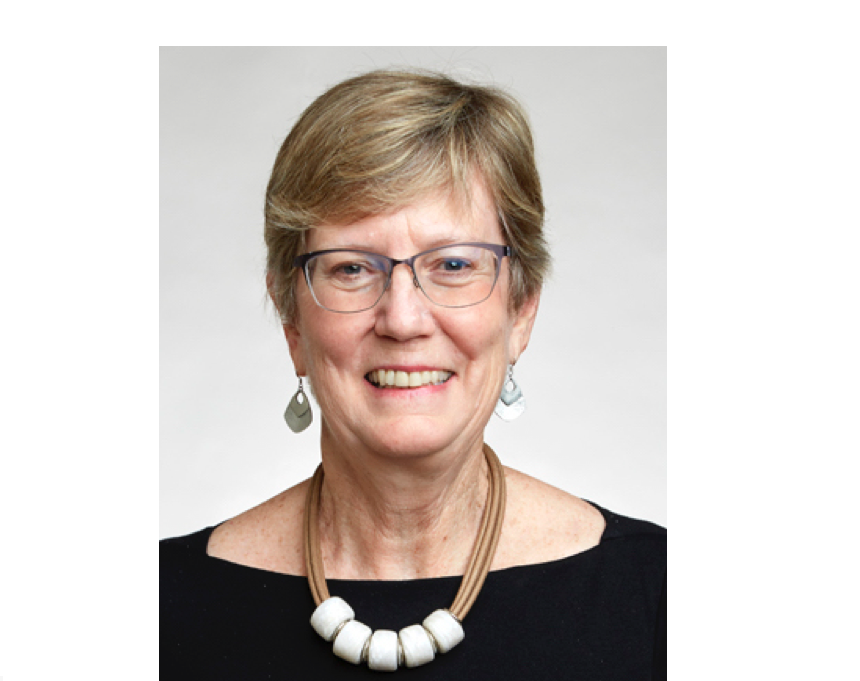
Nancy Reid
University of Toronto
Keynote Lectures
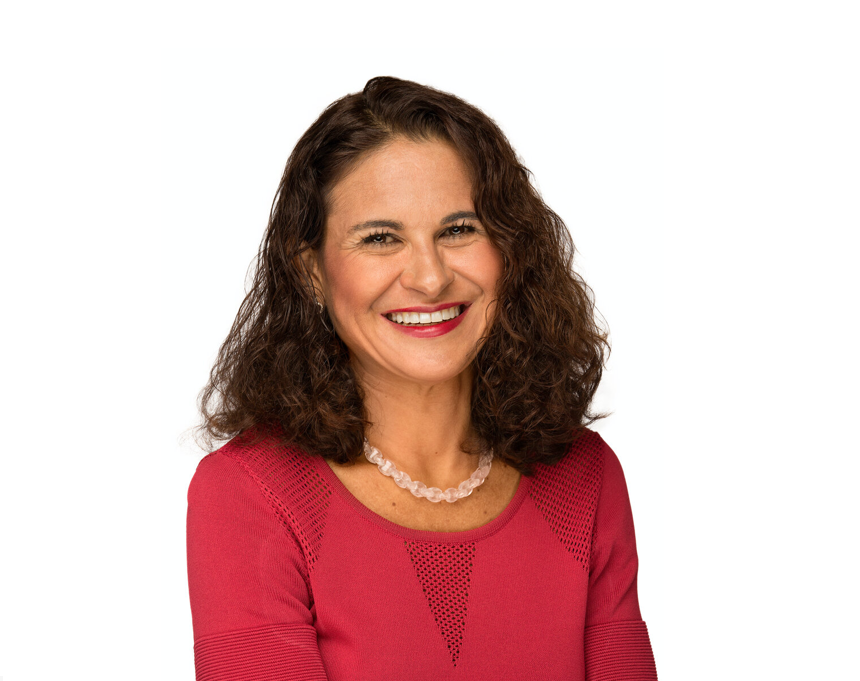
Francesca Dominici
Harvard University
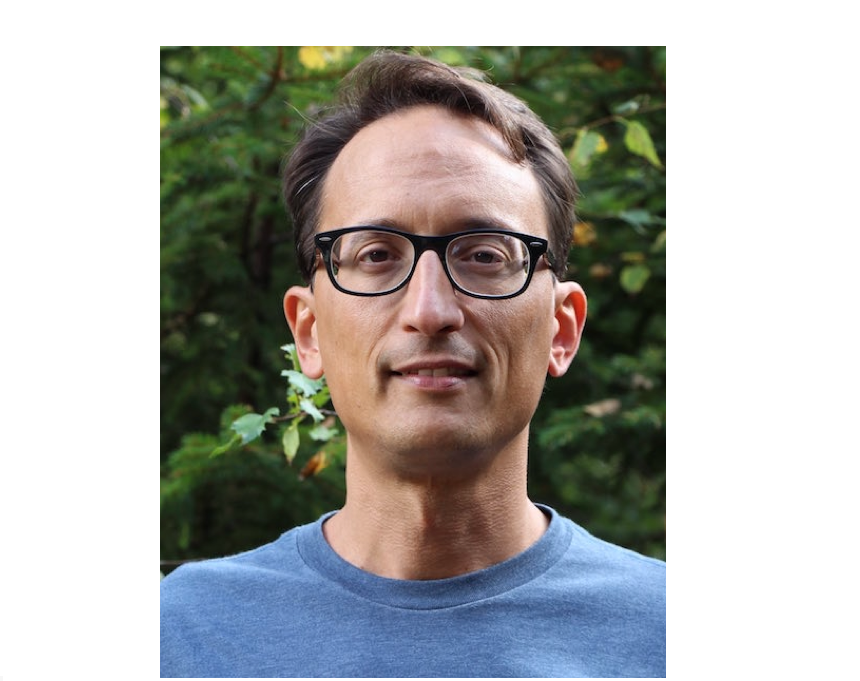
Antonio Lijoi
Bocconi University
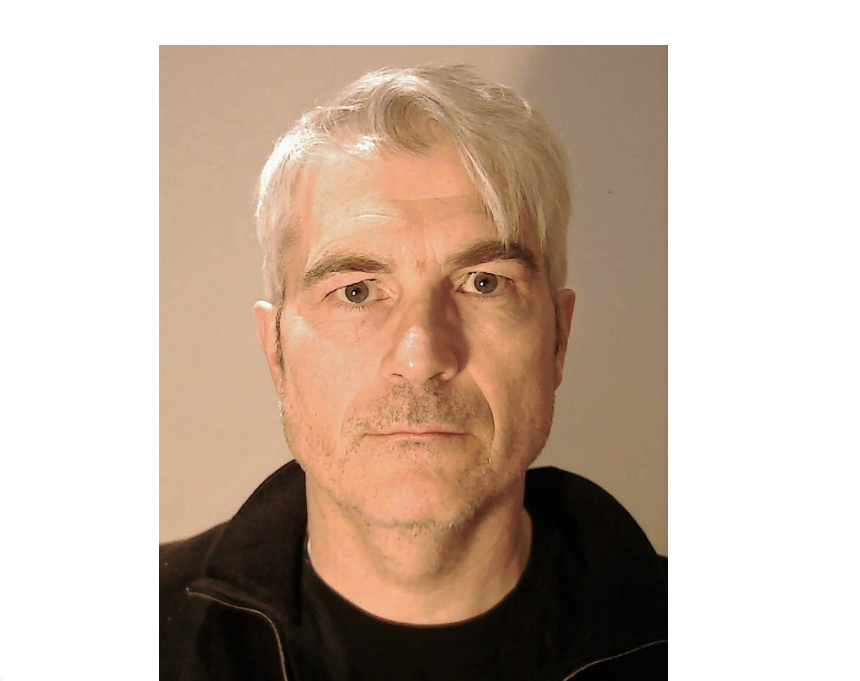
David A. Stephens
McGill University
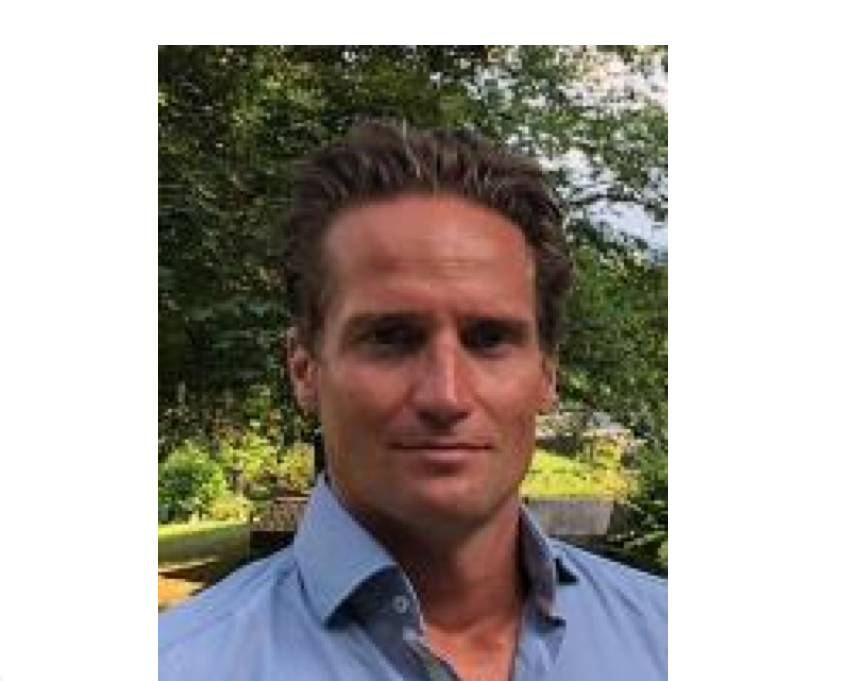
Richard Nickl
University of Cambridge
Bruno de Finetti Lecture
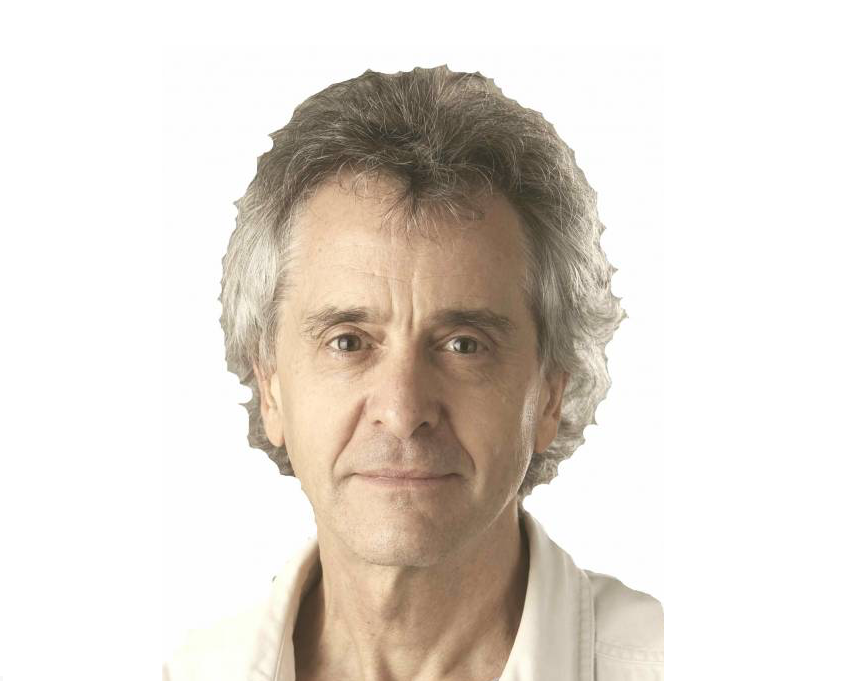
Mike West
Duke University
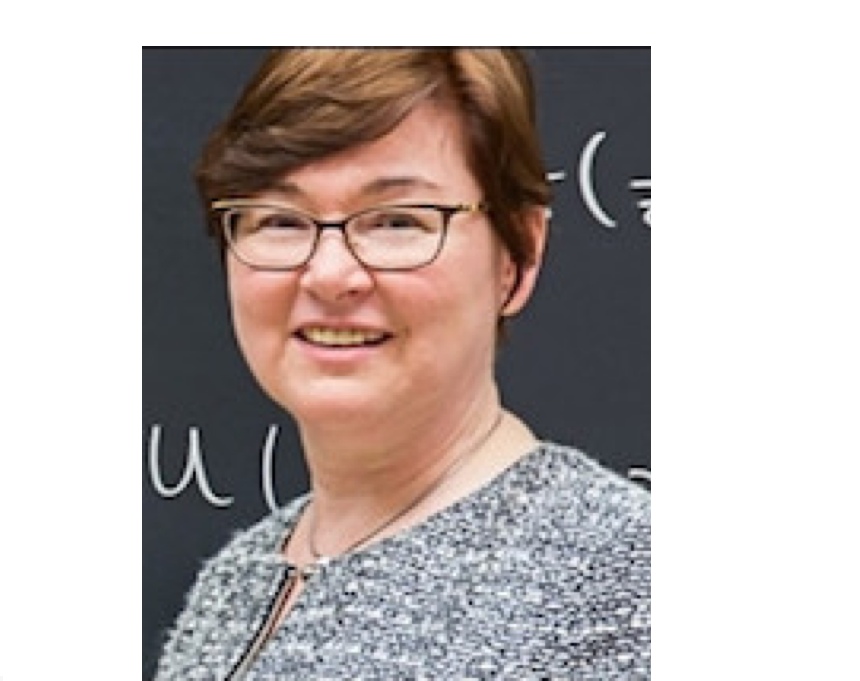
Discussant: Sylvia Frühwirth-Schnatter
Vienna University of Economics and Business
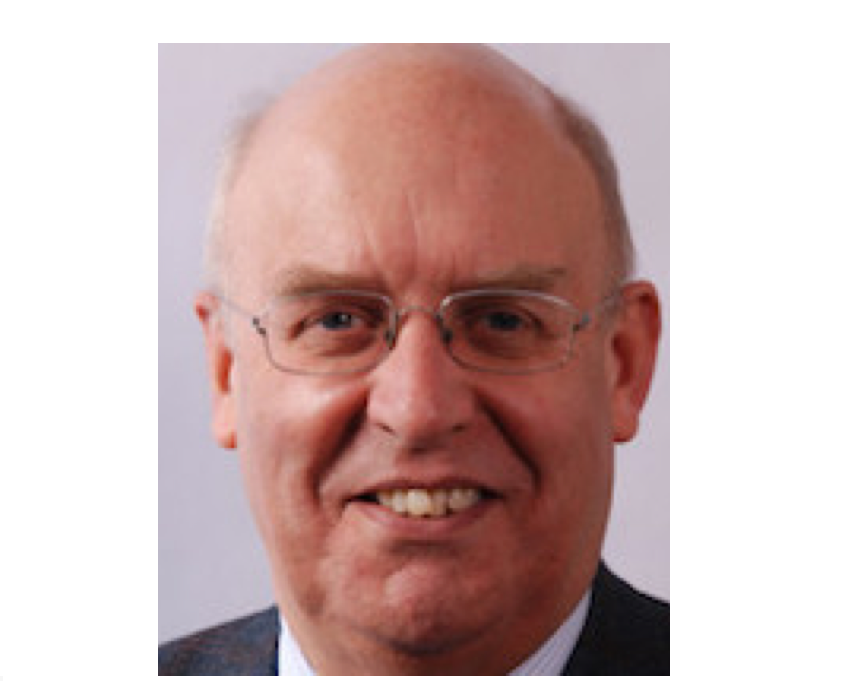
Discussant: Herman van Dijk
Econometric Institute, Erasmus University Rotterdam
Susie Bayarri Lecture
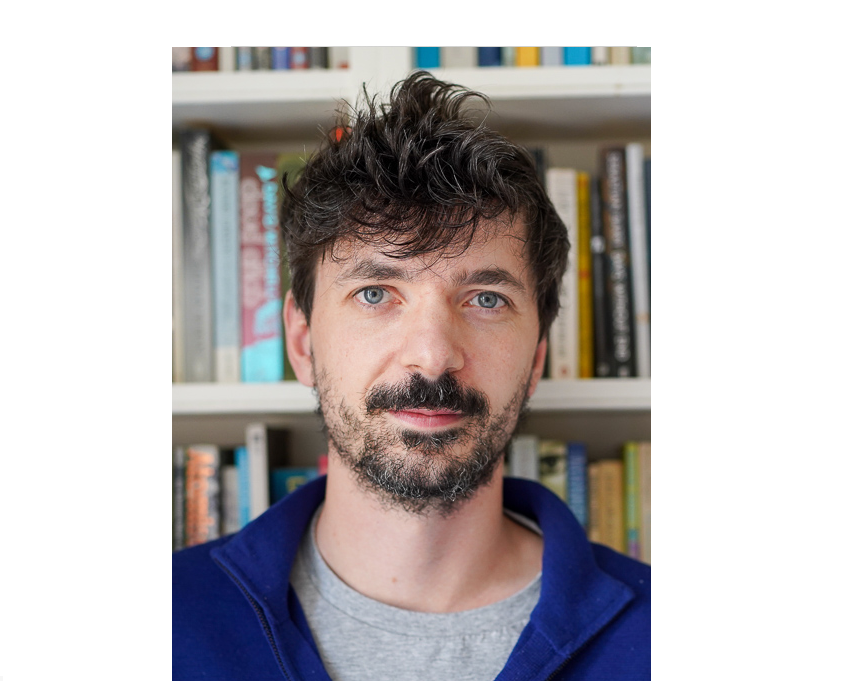
Pierre Jacob
ESSEC Business School
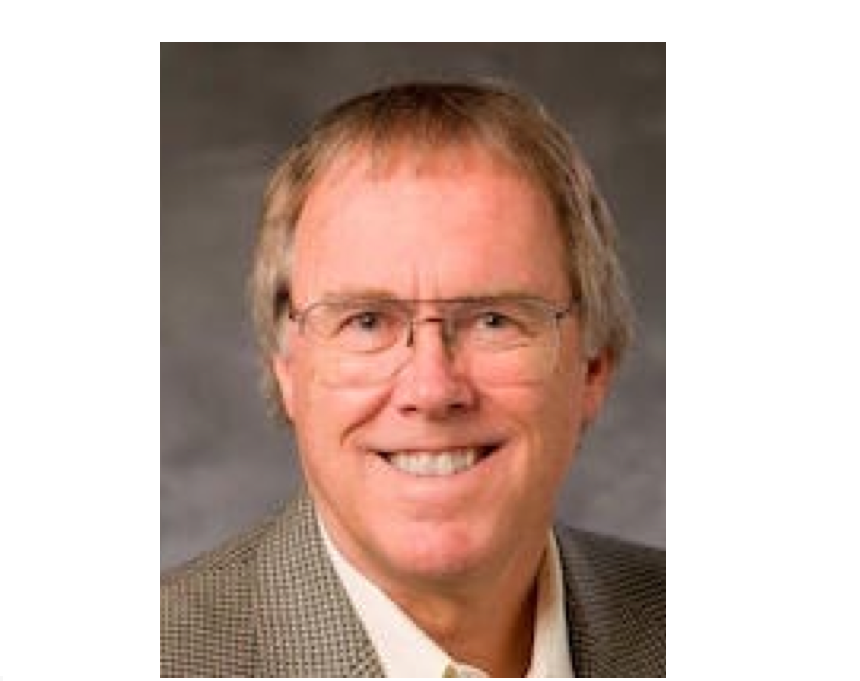
Discussant: Jim Berger
Duke University
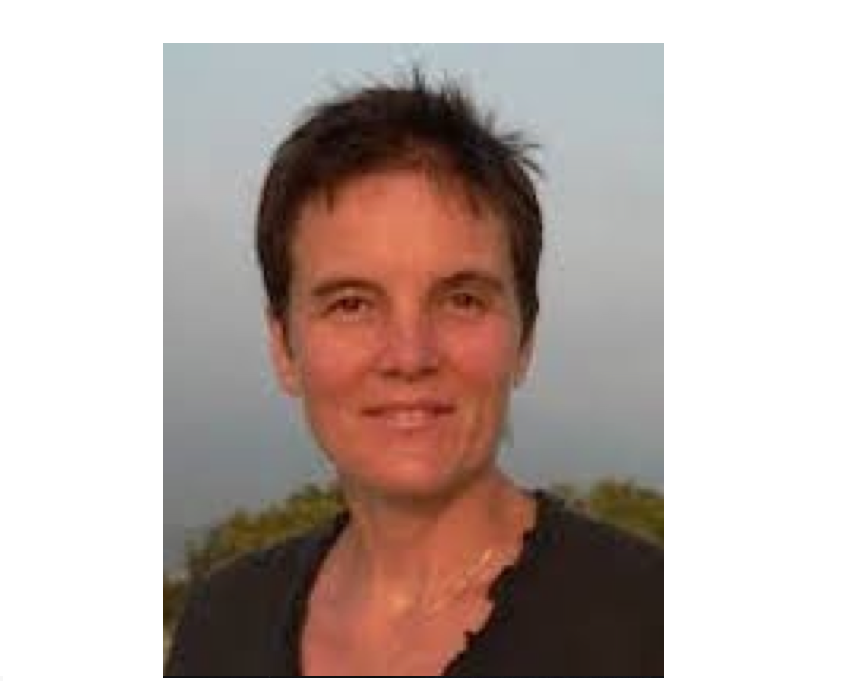
Discussant: Judith Rousseau
University of Oxford
The Program
The ISBA 2022 event schedule
Click here to view the Plenary, Invited Sessions, Contributed Talks, and Poster Sessions
Local Information
Event venue and accomodation
Hotel Bonaventure, Montreal, Canada
Iconic landmark of the city, the Bonaventure offers elegance in a unique setting in Montreal. Winner of several awards for its high level of quality and service, the hotel continues to maintain its distinctive personality and character.
Transportation
Montréal-Pierre Elliott Trudeau International Airport
Located 20 kilometres from downtown Montréal, with multiple highway accesses, Montreal-Trudeau has recently completed a vast expansion and modernization program. Its world-class air terminal is designed to provide you with the most efficient, comfortable and safe airport experience possible. Equipped with the latest airport technologies, it also offers a comprehensive range of services to suit all tastes and budgets.
Sponsors
Platinum Level
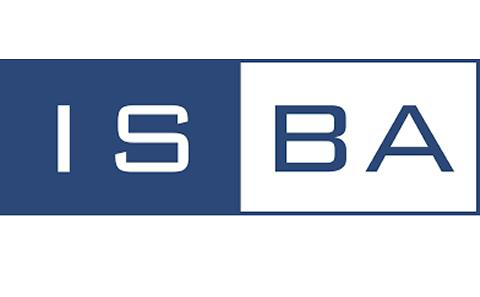
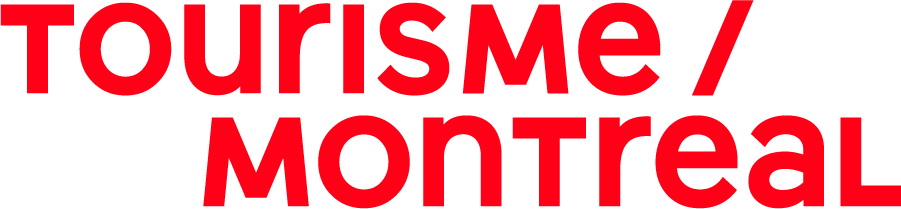
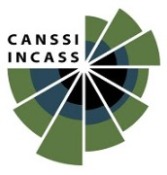
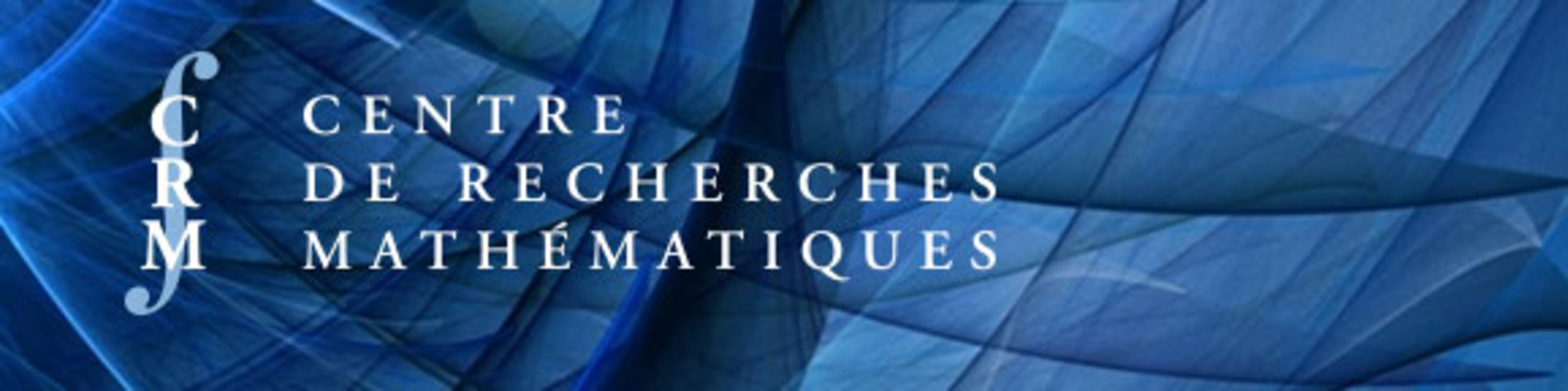
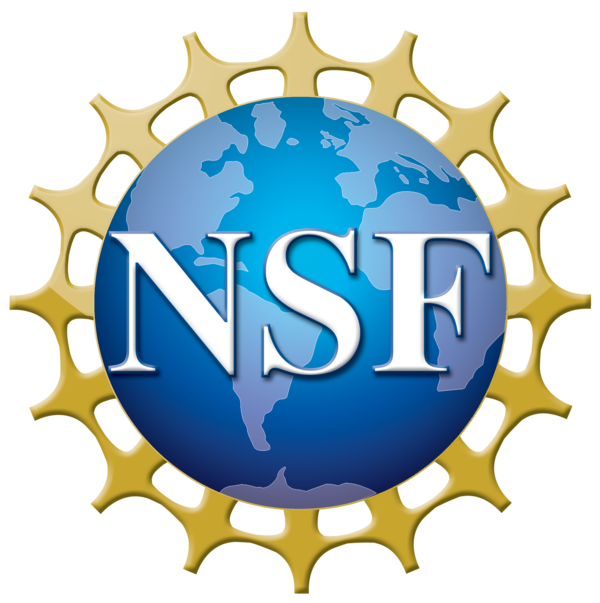
Gold Level
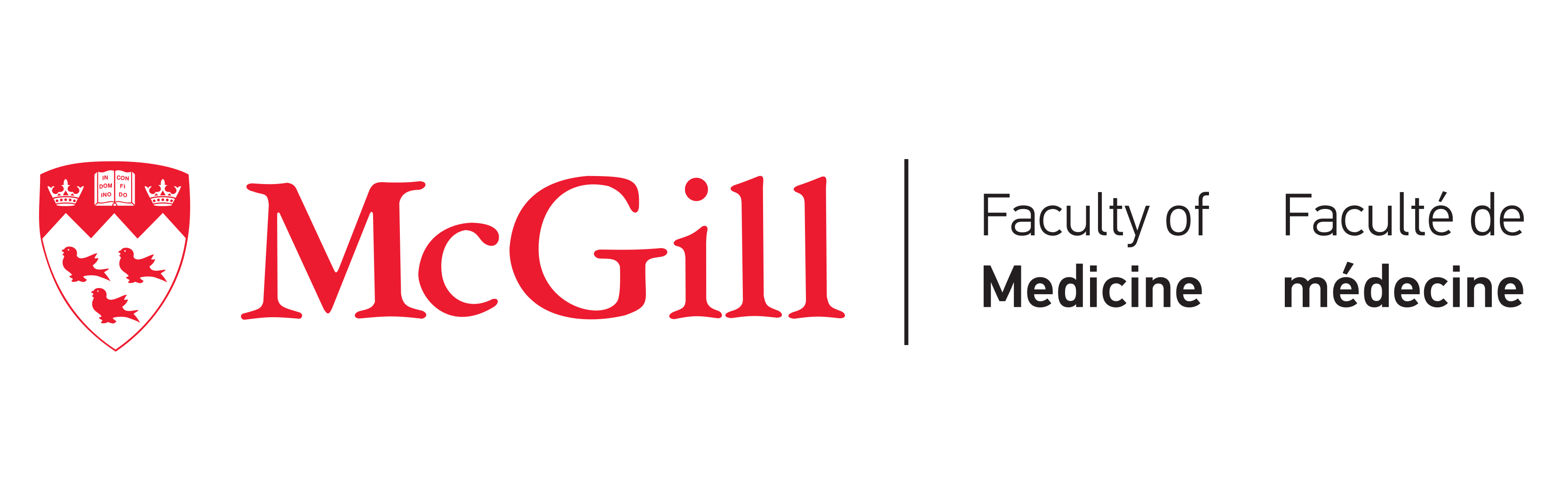
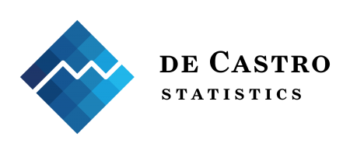
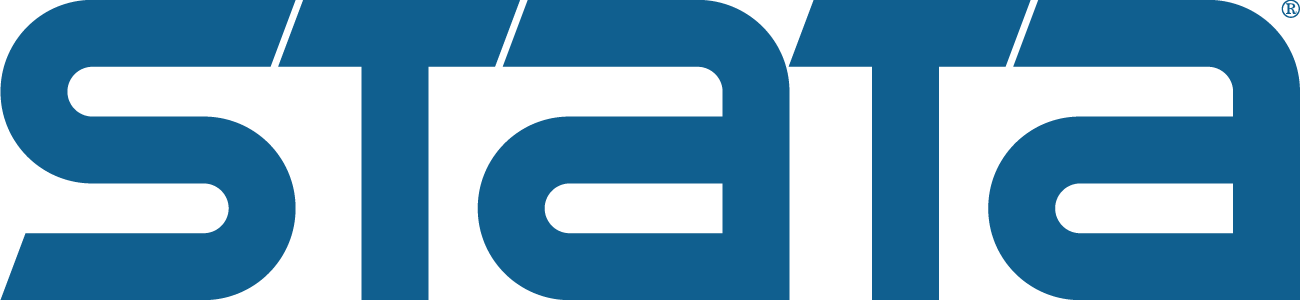

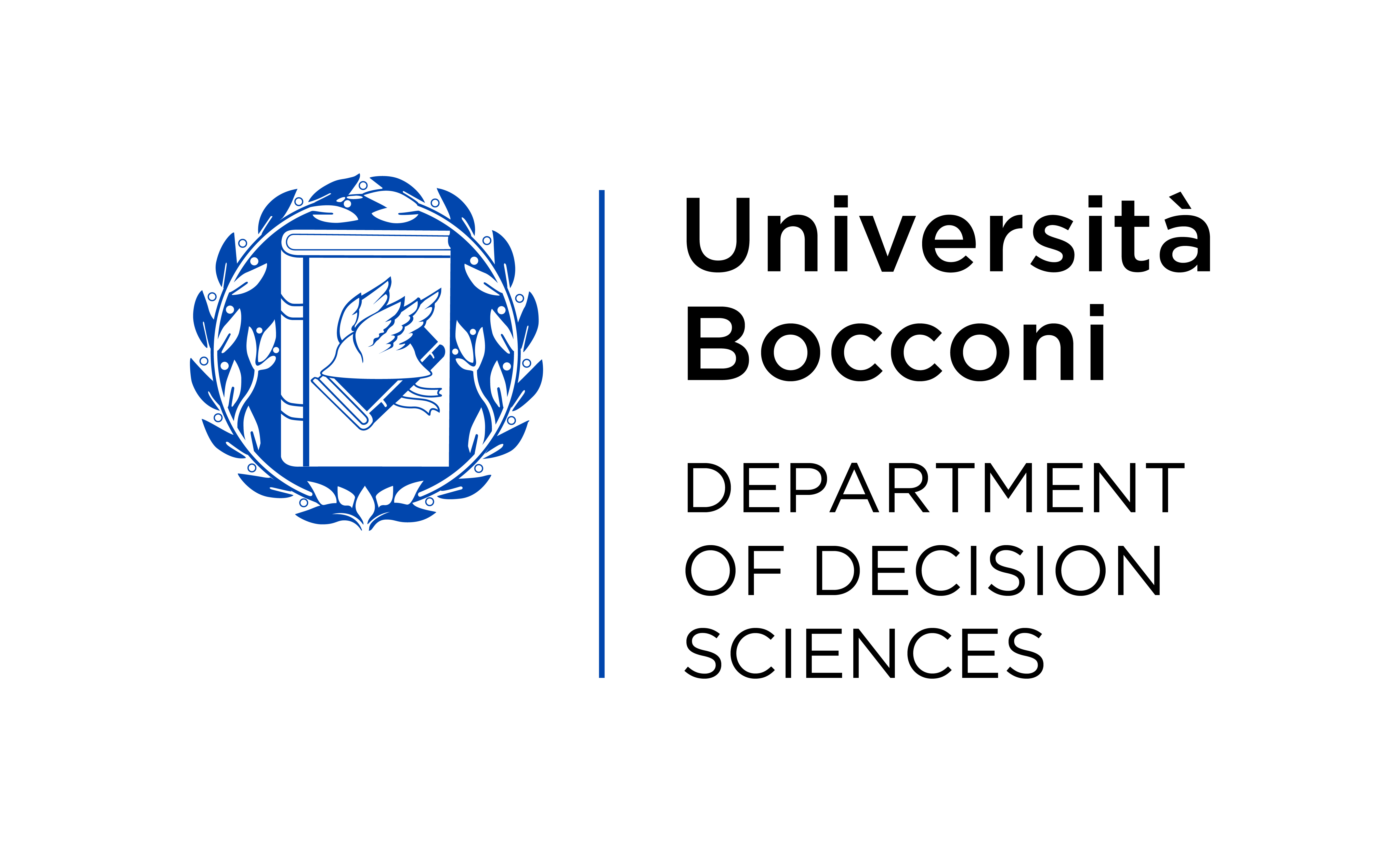
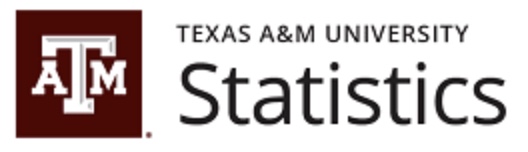
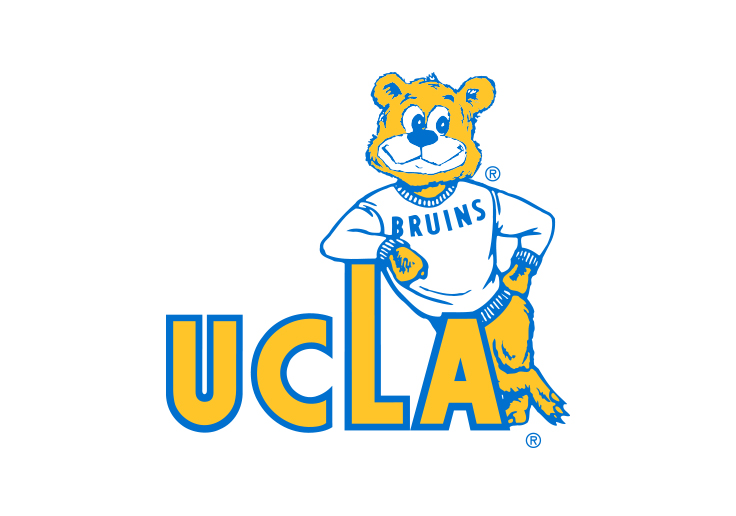
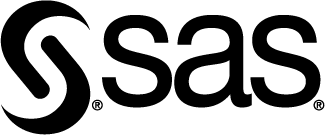
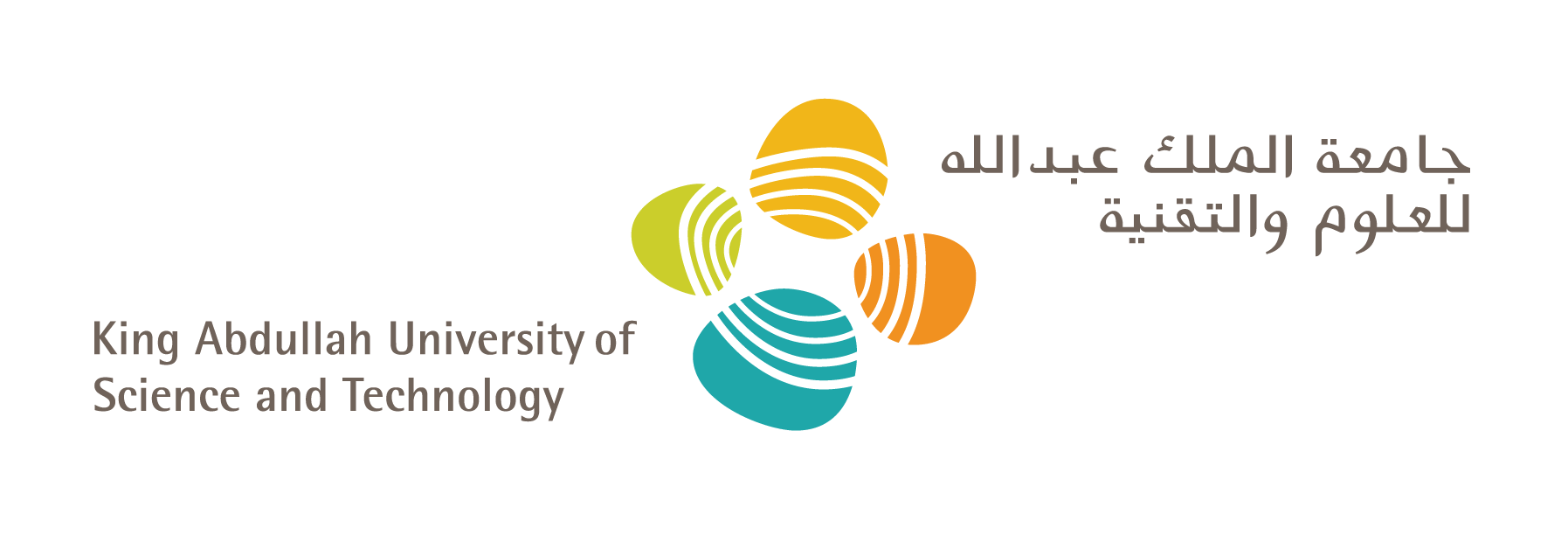
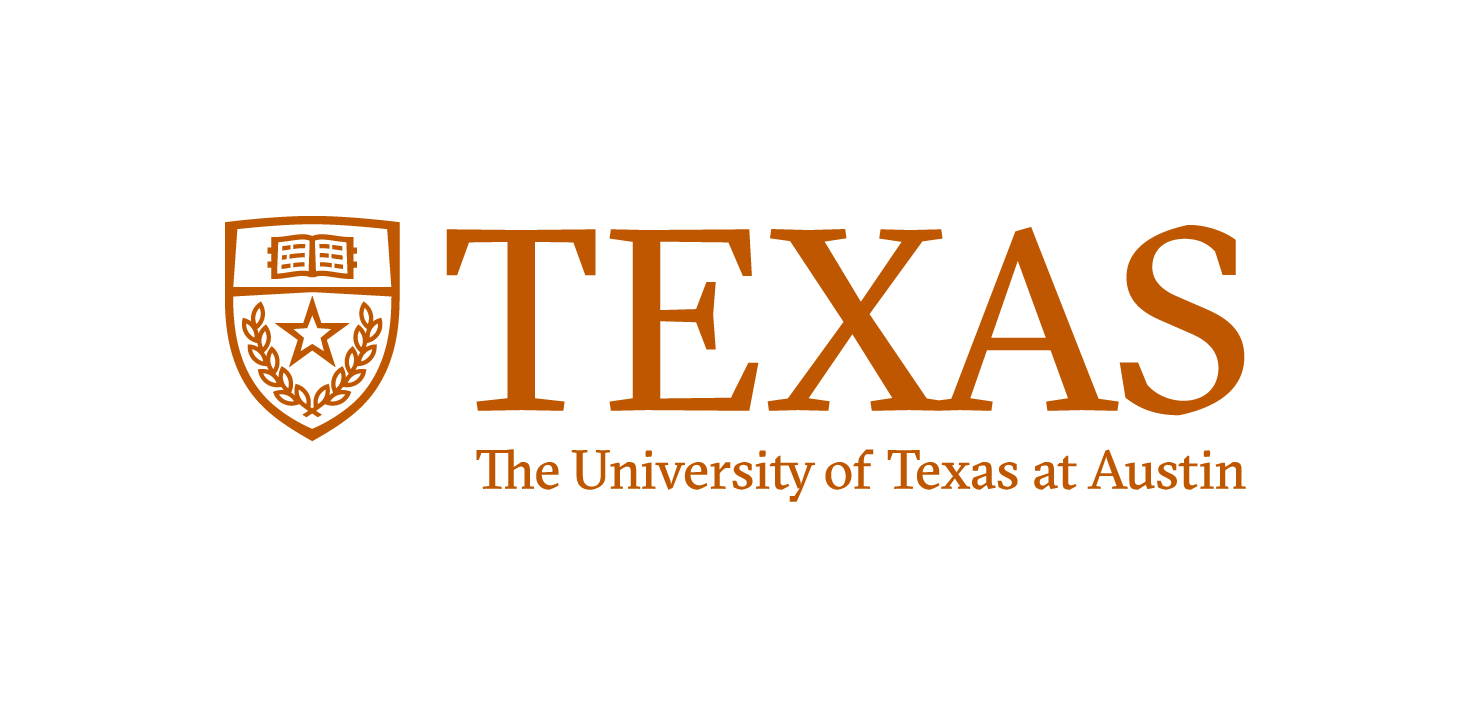
Silver Level
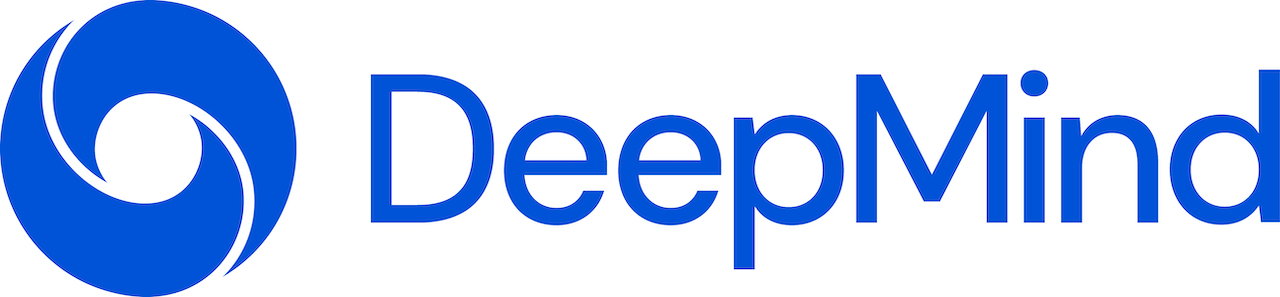
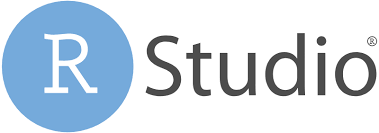
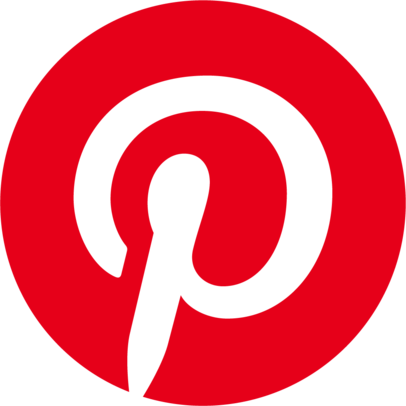
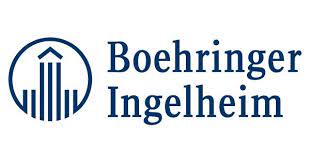
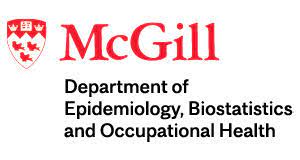
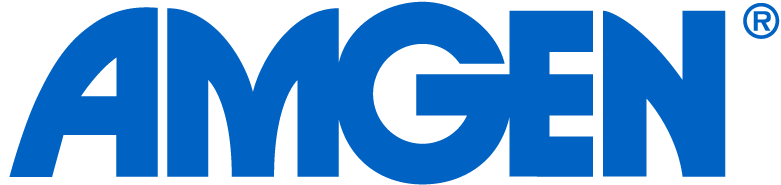
Bronze Level
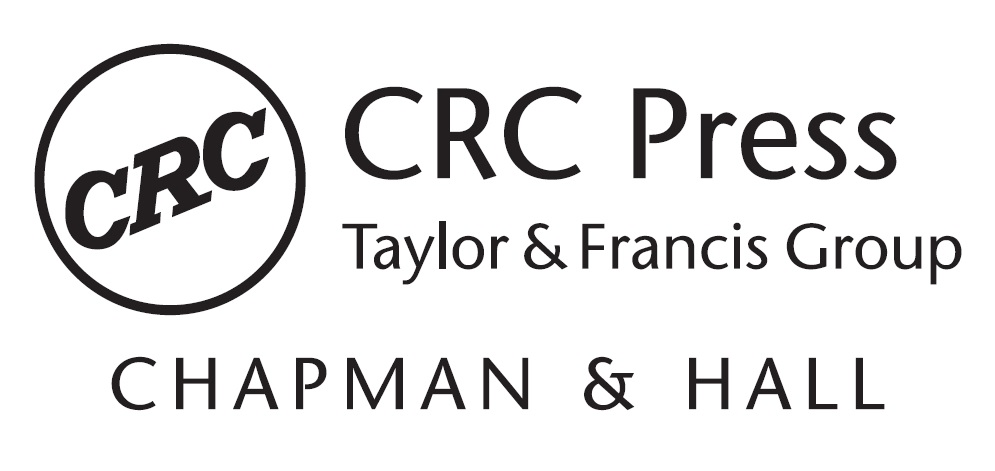
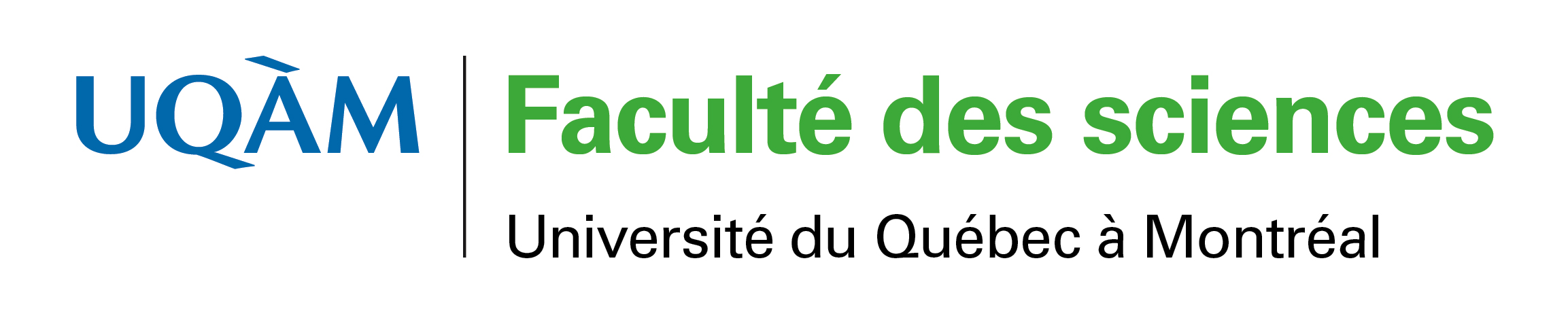
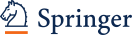
F.A.Q.
-
What is the size of the poster boards? Where to print my poster in Montreal?
Each poster board is 45 inches wide and 91 inches tall. Please see the following document for more details: Poster Board Details
-
How do I get a letter to apply for a VISA to travel to Canada?
Please send an email isba2022loc@gmail.com providing your name as it reads in your passport. If you are giving a presentation provide title and type (invited talk, contributed talk or poster) of your presentation.
-
Are there discounted room rates for the hotel?
ISBA blocked a number of rooms at the Hotel Bonaventure at special rates for those attending the meeting. For more information, please see the following link:
-
How can I participate at ISBA?
Check the "news" section of this website for updates on deadlines to submit proposals, abstracts, and materials for ISBA.
-
What is the closest airport to the conference venue?
Montréal-Pierre Elliott Trudeau International Airport is the closest airport to the conference at 19.2 km (11.9 miles) away.
-
What is the impact of COVID-19 on the conference?
The conference will adhere to the follow the COVID-19 guidelines that are being enforced in Montreal, Canada. Some of the current guidances can be found here:
Contact Us
Drop us a line if you have any questions. Requests for customized receipts or invoices are subject to a $25 USD administrative fee. Please pay your fee at https://bayesian.org/civicrm/event/register/?reset=1&id=51 and contact us at isba2022@bayesian.org with the details for your request.